The BiO-Nets
- 1University of Sydney
- 2University of New South Wales
- 3Electrical and Computer Engineering, University of Pittsburgh, USA
- 4JD Finance America Corporation, Mountain View, CA, USA
Our Works
BiO-Net: Learning Recurrent Bi-directional Connections for Encoder-decoder Architecture (MICCAI2020)
Tiange Xiang1, Chaoyi Zhang1, Dongnan Liu1, Yang Song2, Heng Huang3,4, and Weidong Cai1.
BiX-NAS: Searching Efficient Bi-directional Architecture for Medical Image Segmentation (MICCAI2021)
Xinyi Wang1,*,
Tiange Xiang1,*,
Chaoyi Zhang1,
Yang Song2,
Dongnan Liu1,
Heng Huang3,4, and
Weidong Cai1.
(* Equal first-author contributions)
Towards Bi-directional Skip Connections in Encoder-Decoder Architectures and Beyond (MIA2022)
Tiange Xiang1,
Chaoyi Zhang1,
Xinyi Wang1,
Yang Song2,
Dongnan Liu1,
Heng Huang3,4, and
Weidong Cai1.
Overview
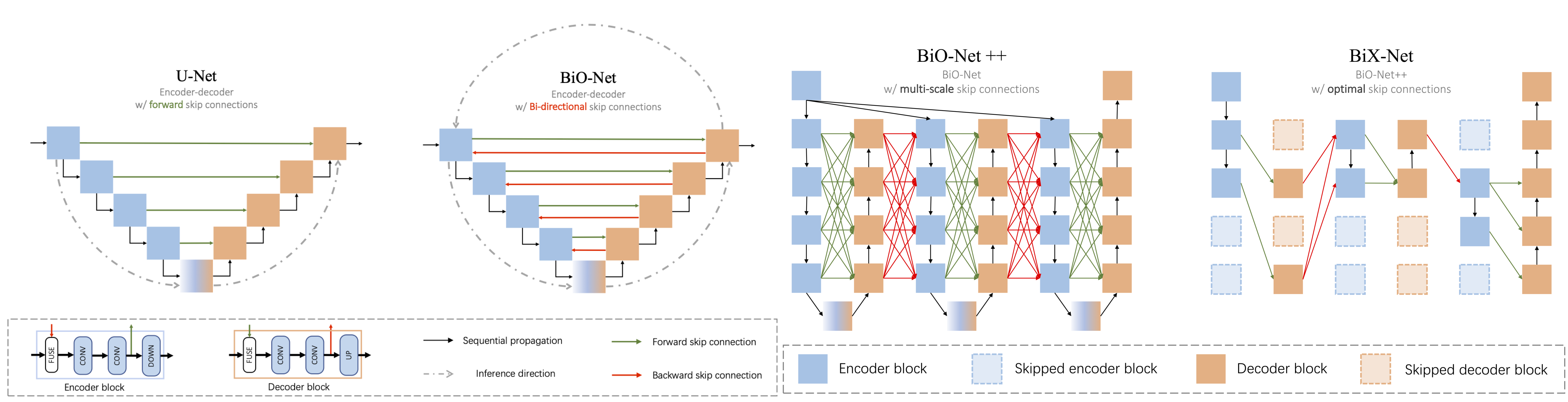
BiO-Net. In this paper, we present a novel Bi-directional O-shape network (BiO-Net) that reuses the building blocks in a recurrent manner without introducing any extra parameters. Our proposed bi-directional skip connections can be directly adopted into any encoder-decoder architecture to further enhance its capabilities in various task domains. We evaluated our method on various medical image analysis tasks and the results show that our BiO-Net significantly outperforms the vanilla U-Net as well as other state-of-the-art methods.
BiX-NAS. In this work, we study a multi-scale (abstracted as 'X') upgrade of a bi-directional skip connected network and then automatically discover an efficient architecture by a novel two-phase Neural Architecture Search (NAS) algorithm, namely BiX-NAS. Our proposed method reduces the network computational cost by sifting out ineffective multi-scale features at different levels and iterations. We evaluate BiX-NAS on two segmentation tasks using three different medical image datasets, and the experimental results show that our BiX-NAS searched architecture (BiX-Net) achieves the state-of-the-art performance with significantly lower computational cost.
Highlights
BiO-Net
- Present a compact substitute of U-Net with paired forward and backward skip connections.
- Recursed to reuse the parameters during training and inference.
- State-of-the-art mIoU (0.704) and DICE (0.825) on MoNuSeg without introducing auxiliary parameters.
BiX-NAS
- Present a multi-scale upgrade of BiO-Net++ following the recurrent bi-directional paradigm.
- Design an effective two-phase Neural Architecture Search (NAS) strategy overcoming the searching deficiency, namely BiX-NAS.
- State-of-the-art mIoU and DICE on three medical image datasets with significantly lower computational cost (0.38M parameters, 28.00G MACs), and the searched BiX-Net will be avaialble in our codebase for comparison.
Qualitative Results
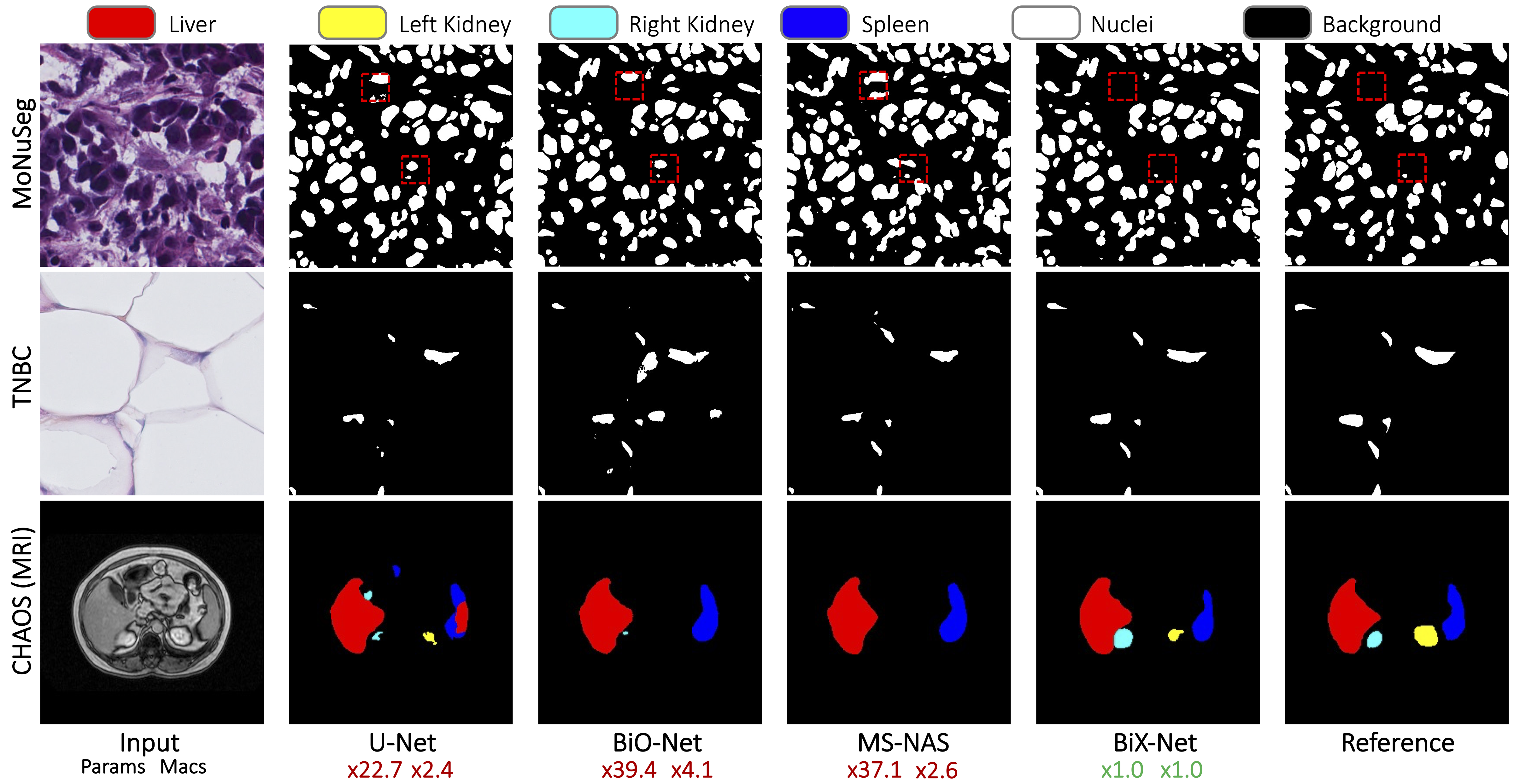
Video
BibTeX
If you find our data or project useful in your research, please cite:@inproceedings{xiang2020bio, title={BiO-Net: Learning Recurrent Bi-directional Connections for Encoder-Decoder Architecture}, author={Xiang, Tiange and Zhang, Chaoyi and Liu, Dongnan and Song, Yang and Huang, Heng and Cai, Weidong}, booktitle={International Conference on Medical Image Computing and Computer-Assisted Intervention (MICCAI)}, pages={74--84}, year={2020}, organization={Springer} } @inproceedings{wang2021bix, title={BiX-NAS: Searching efficient bi-directional architecture for medical image segmentation}, author={Wang, Xinyi and Xiang, Tiange and Zhang, Chaoyi and Song, Yang and Liu, Dongnan and Huang, Heng and Cai, Weidong}, booktitle={International Conference on Medical Image Computing and Computer-Assisted Intervention}, pages={229--238}, year={2021}, organization={Springer} } @inproceedings{xiang2022bio, title={Towards Bi-directional Skip Connections in Encoder-Decoder Architectures and Beyond}, author={Xiang, Tiange and Zhang, Chaoyi and Wang, Xinyi and and Song, Yang and Liu, Dongnan and Huang, Heng and Cai, Weidong}, booktitle={Medical Image Analysis}, year={2022}, organization={Springer} }